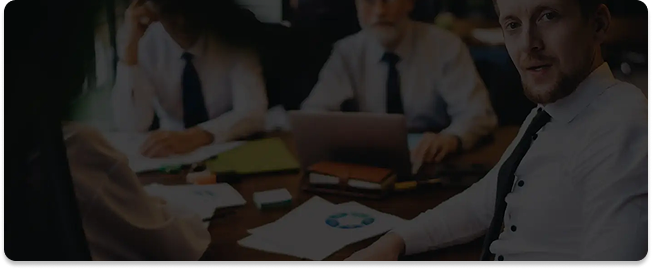
Customer.com
Customer.com is exclusively for sale at Brannans. A highly in-demand premium name, this domain won't stay on the market for long. Whether you want to boost your brand or invest in a strong digital asset, Customer.com is sure to help you achieve your goals.
Contact Us
Have questions? Get in touch and a member of our team will respond shortly.
Domain Characteristics
Potential Applications
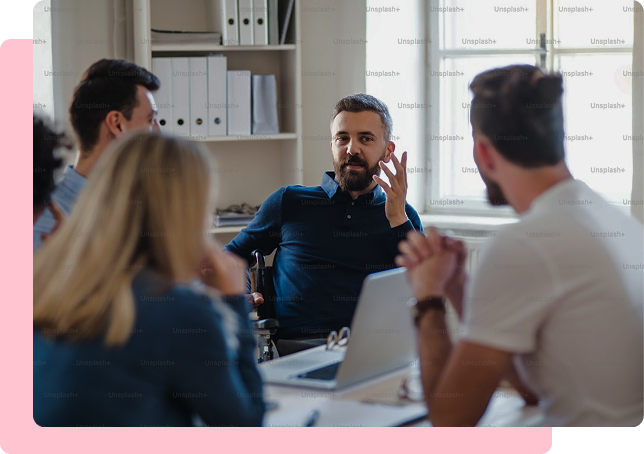
Why Choose Brannans?
With decades of experience in ultra-premium domain name sales, Brannans is the brokerage of choice for one-word .COM domain names.
We have helped countless organizations secure their domain of choice, from Fortune 500 companies to VC-backed startups.
Our approach is confidential, secure and bespoke. Our team are on hand to offer support at any time and answer any questions you may have.
Frequently Asked Questions
Below is an example of some of our frequently asked questions for domain buying.
If you have a question that isn't covered here, please contact us today.